Edge Computing
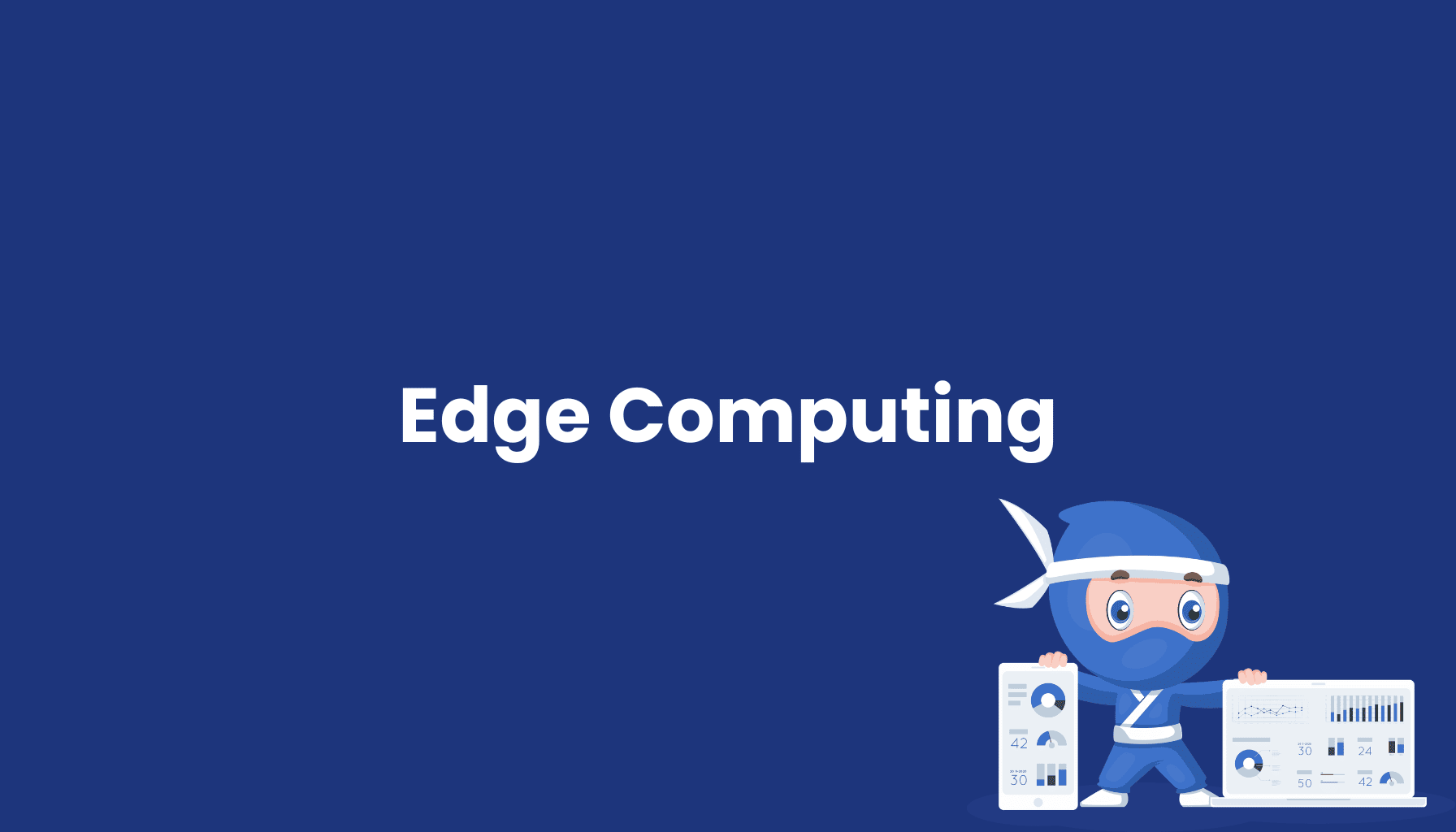
What is Edge Computing?
Edge Computing is a decentralized data processing architecture where data is collected, analyzed, and processed closer to its source or "edge" of the network, rather than being sent to a central data center or cloud for processing. This approach allows applications to access and act on local data in real-time, enhancing response times and reducing network load.
Advantages of Edge Computing
- Reduced Latency: Processing data close to its source minimizes delays, enabling rapid response times, which is essential for time-sensitive applications.
- Optimized Bandwidth Usage: Local data processing reduces the amount of data sent to central locations, alleviating network congestion.
- Cost Savings: Lowered data transmission needs result in reduced costs associated with data transfer and network usage.
- Enhanced Resilience: Decentralized processing means systems often continue to function even in case of network interruptions.
Key Applications of Edge Computing
Edge Computing is widely adopted across various sectors to enable real-time analysis and decisions directly at data collection points. Key applications include:
- Autonomous Vehicles: Real-time data analysis for safe navigation and immediate responses to road conditions.
- Smart Cities: Real-time monitoring and management of city infrastructure, such as traffic and energy systems, for optimized urban living.
- Industry 4.0: Edge Computing facilitates real-time production data analysis to streamline and improve production processes instantly.
Role of Edge Computing in Industry 4.0
In Industry 4.0, Edge Computing is integral as modern manufacturing relies heavily on real-time data to maximize efficiency. Processing data at the source allows businesses to quickly adapt to production changes, increasing flexibility and productivity. This provides a competitive advantage through faster responses to changing market demands.
Technological Foundations of Edge Computing
Edge Computing derives its power from modern technological integrations such as:
- Artificial Intelligence (AI): AI algorithms run locally to analyze data and make automated, real-time decisions.
- Machine Learning (ML): ML models analyze historical and current data to continuously improve processes and adapt dynamically.
- IoT Sensors and Actuators: These capture data from physical processes, enabling direct interaction with on-site operations.
Challenges and Solutions in Edge Computing
Implementing Edge Computing brings certain challenges that require targeted solutions:
- Security Requirements: Processing sensitive data locally demands robust security to ensure data integrity and protection.
- Complex Integration: Seamlessly integrating Edge Computing into existing IT infrastructures can be complex and needs strategic planning.
- Hardware Costs: Deploying and maintaining Edge devices may initially require higher investment, though these costs are offset by process efficiencies and long-term savings.
Conclusion
Edge Computing is essential for data-intensive, real-time applications. By processing data at its source, it reduces latency, optimizes bandwidth, and saves on data transfer costs. Edge Computing is especially valuable in connected environments such as Industry 4.0, autonomous vehicles, and smart cities, where real-time, reliable decision-making is crucial.